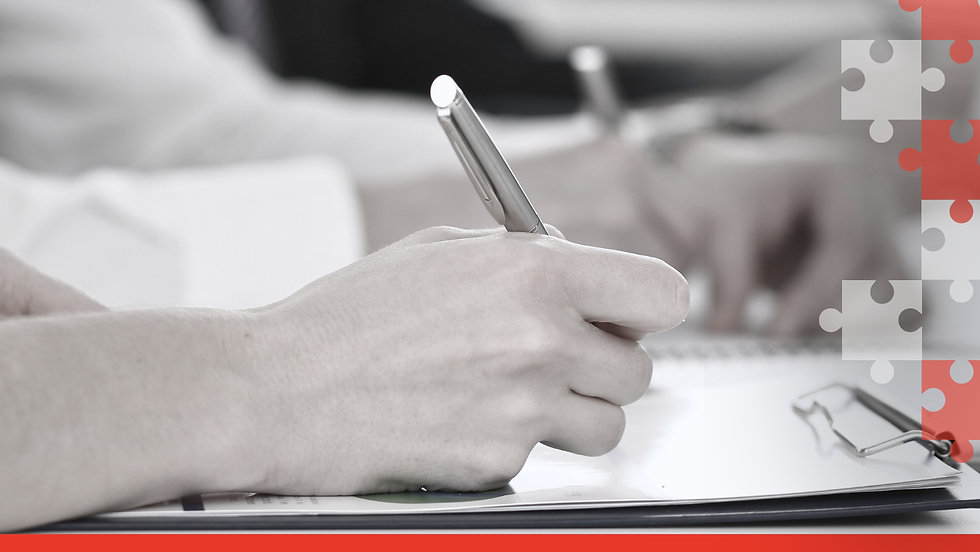
by Basia Walczak, Manager, Privacy and Michael Pascu, Senior Manager, Artificial Intelligence
As artificial intelligence (“AI”) becomes a core part of business operations, understanding the associated risks is crucial. According to a recent survey, 65% of organizations have prioritized the evaluation of AI risk using their existing internal processes, and 55% are relying on current and pending laws to assess AI-related risks.¹ As AI adoption grows, it is more important than ever to identify potential issues and establish safeguards to ensure AI is used responsibly and effectively.
This article discusses the main steps organizations should take to identify and mitigate AI-related risks via an AI risk assessment. We highlight key AI risks to consider when conducting an AI risk assessment and suggest a process for undertaking this assessment.
What is an AI risk assessment?
An AI risk assessment involves evaluating the potential adverse outcomes of deploying AI systems, including ethical, legal, operational, and technical risks. The goal of the AI risk assessment is to proactively identify and mitigate these risks while maximizing the benefits AI can bring to your business. Without appropriate care, AI could lead to privacy issues, reputational damage, or discriminatory outcomes.²
AI risk assessments help businesses identify and address potential risks, ensure regulatory compliance, protect against data privacy breaches and eliminate biases that could result in unfair outcomes. By proactively managing these risks, businesses can build public trust, safeguard their reputation, and avoid legal liabilities.
What risks should be considered?
There are various AI-related risks that organizations should consider when evaluating their AI systems.³ These include:
Validity and reliability: AI systems should fulfill their intended purpose, produce consistent results, not fail or break unexpectedly, be accurate, and respond appropriately to unexpected situations.
Accountability and transparency: Clearly define roles and responsibilities for everyone involved from design and deployment of the AI system. Whenever possible, share information about how the AI system operates and the data it was developed with in ways that impacted audiences can understand.
Explainability: Provide clear explanations and reasoning behind the AI system’s decisions, predictions, or recommendations.
Security and resiliency: Ensure AI systems are resistant to adversarial attacks, such as data poisoning and data or intellectual property theft. They should also be able to return to normal functionality after an adverse event.
Safety: AI systems should avoid negatively impacting humans, property, or the environment. They should allow for human intervention and be capable of being shut down as needed.
Bias and non-discrimination: AI systems should be accessible to all individuals. Assess the AI system’s performance and implement measures to manage and mitigate harmful discrimination, preventing the exacerbation of existing social biases.
Privacy: Uphold human autonomy, identity, and dignity. Ensure the AI system’s measures for protecting personal and data privacy comply with applicable laws.
When and how should you conduct an AI risk assessment?
An AI risk assessment should be conducted during the early stages of design and development. This allows for risks to be mitigated during the system’s design and validation, before deployment. Below are the key steps involved in each stage of an AI system’s lifecycle, with a particular emphasis on conducting an AI risk assessment.
Stage of the AI System | Key Activities |
1. Ideation and Feasibility |
|
2. Solution Design |
|
2A. AI Risk Assessment |
|
3. Solution Development, Testing, and Validation |
|
4. Deployment and Monitoring |
|
Conclusion
AI offers immense potential for business innovation and efficiency. However, the associated risks require careful management. By structuring a thorough AI risk assessment, businesses can navigate the complexities of AI deployment responsibly, ensuring that their AI initiatives are both beneficial and secure. Prioritize transparency, stakeholder involvement, and continuous monitoring to build a robust AI risk management framework.
How can we help?
INQ’s portfolio of AI services is customized to fit your specific needs and get you AI-ready. To learn more, visit our website at www.inq.consulting or contact us at ai@inq.consulting. To keep up with the latest in AI news, subscribe to the Think INQ newsletter.
¹ AuditBoard, Digital Risk Report, 2024
² Wharton, University of Pennsylvania, “Artificial Intelligence Risk & Governance,” Artificial Intelligence/Machine Learning Risk & Security Working Group (AIRS), online < https://ai.wharton.upenn.edu/white-paper/artificial-intelligence-risk-governance/ >.
³ These risks have been adapted from those discussed in the NIST AI Risk Management Framework.
Comments